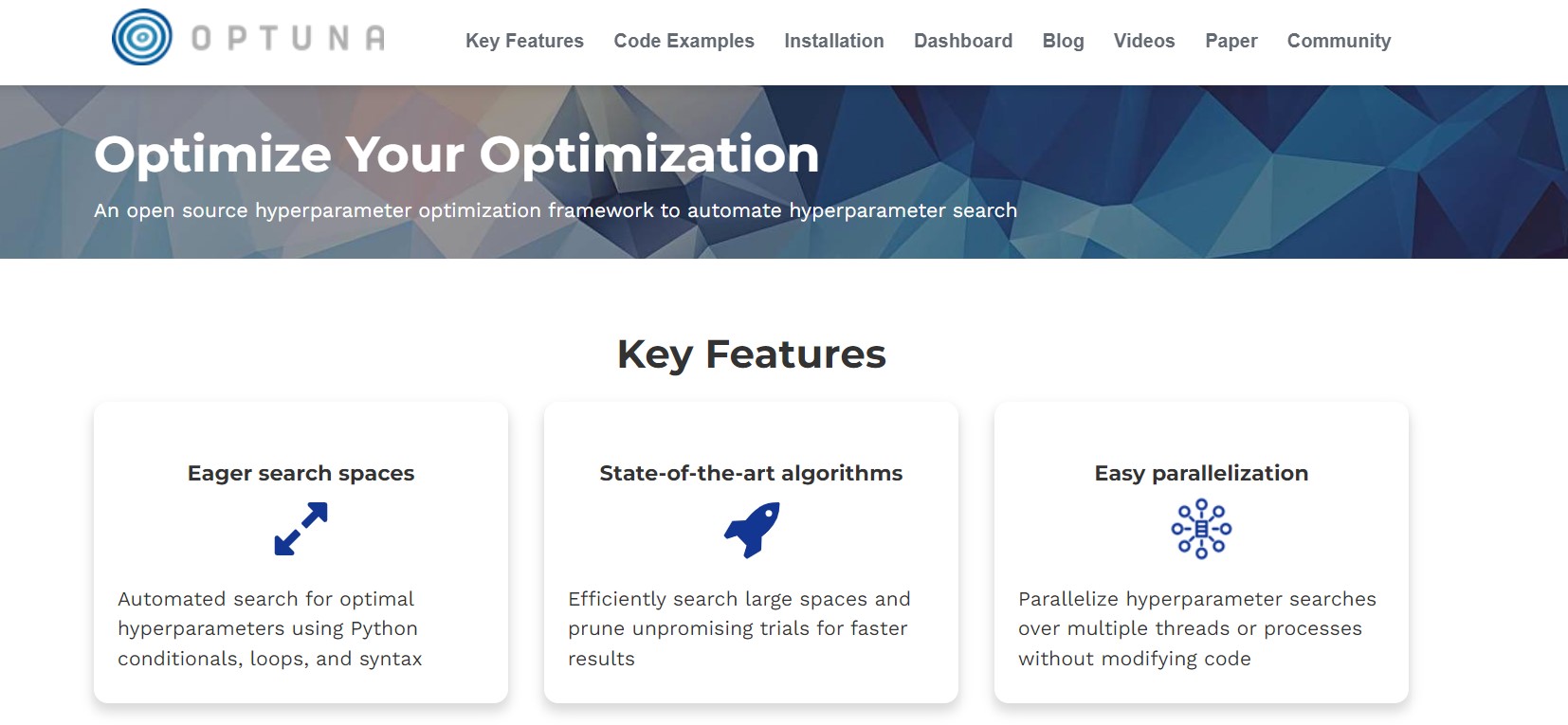
Optuna: The Ultimate Hyperparameter Optimization Tool
Machine learning models have become an integral part of various industries, from healthcare to finance. But building a high-performing model requires a lot of effort and experimentation, especially when it comes to choosing the right hyperparameters. That's where Optuna comes in, an open-source hyperparameter optimization framework that automates the process of finding the best set of hyperparameters for your model. In this blog, we'll dive deep into Optuna's capabilities and features, including its unique strategies like sampling and pruning mechanisms, speed and parallelization benefits, and hands-on code implementation examples. Don't let hyperparameter optimization slow you down - discover why Optuna is the ultimate tool for optimizing machine learning models.
Understanding Hyperparameter Optimization
Hyperparameter optimization is crucial for enhancing the performance of machine learning models. Optuna offers advanced optimization techniques to efficiently explore the hyperparameter space. By defining your objective function, Optuna can automatically find the best hyperparameters for you. This automation saves both time and effort. With Optuna, you can achieve superior results with your machine learning models.
The Importance of Hyperparameter Optimization in Machine Learning
Hyperparameter optimization plays a crucial role in achieving optimal performance in machine learning models. Properly tuned hyperparameters can significantly improve the accuracy and generalization of your models. Optuna's optimization methods, like TPE and random search, help explore the search space effectively. Through hyperparameter optimization, you can find the best configuration for your model, improving its performance. Optuna's objective value visualization allows you to track and analyze the progress of your hyperparameter optimization.
Introduction to Optuna
Optuna, a next-generation hyperparameter optimization framework, is an open-source library developed by Takuya Akiba and his team. Its define-by-run API seamlessly integrates into existing machine learning workflows. With Optuna, you can easily optimize hyperparameters for various machine learning algorithms and models. The framework provides extensive documentation and examples to guide you throughout the optimization process. Optuna saves time and effort by automating the search for the best hyperparameters.
Features and Benefits of Optuna
Optuna offers a wide range of features that make it the ultimate hyperparameter optimization tool. With support for various optimization methods, including TPE and random search, Optuna allows you to define and optimize both continuous and categorical hyperparameters. The automatic pruning of unpromising trials saves computational resources, while the visualization of optimization history helps analyze the importance of each hyperparameter. Additionally, Optuna's study object enables efficient parallel optimization of hyperparameters, ensuring less time is spent on tuning models.
How Does Optuna Work?
Optuna works by exploring the search space of hyperparameters using various optimization methods. It starts with defining an objective function that evaluates the performance of hyperparameter sets. Then, it suggests new configurations based on previous trials and guides the search towards better parameters. Optuna keeps track of the best parameters found so far and their evaluation score.
The Architecture of Optuna
Optuna employs a client-server architecture for hyperparameter optimization. The server handles trial distribution, storage, and communication with the client. On the other hand, the client defines the study object and implements the objective function. This architecture enables distributed optimization across multiple machines or processes. Communication between the client and server is facilitated through a REST API. Optuna's architecture ensures efficient and scalable hyperparameter optimization, allowing users to save time while achieving optimal results.
Diving Deep into Optuna's Capabilities
Optuna caters to a wide range of hyperparameter optimization use cases and supports various machine learning algorithms, such as LightGBM and neural networks. It offers customizable pruning strategies to enhance optimization speed. With its user-friendly API, it seamlessly integrates into existing machine learning pipelines. Optuna's extensive documentation and example repository provide guidance for complex optimization scenarios. Harness the power of Optuna to fine-tune your models and achieve optimal performance.
Evaluation Criteria and Optuna's Performance
Optuna utilizes an evaluation metric, like AUC, to gauge the quality of each trial's hyperparameter configuration. It keeps track of the best parameters discovered throughout the optimization process based on the evaluation metric. Optuna employs optimization methods such as TPE and random search, which efficiently explore the hyperparameter search space. Experimental results have demonstrated that Optuna outperforms other optimization tools in finding superior hyperparameters. Optuna offers optimization history visualizations that aid in analyzing the search process and evaluating its performance.
Ease of Use and API in Optuna
Optuna offers a user-friendly and intuitive API for smooth hyperparameter optimization studies. Its API allows easy definition of the search space for hyperparameters. The study object in Optuna helps manage and track the progress of optimization. GitHub's Optuna repository offers extensive documentation and examples. Additionally, Optuna's API allows customization and integration with other ML frameworks and tools. With Optuna, optimizing hyperparameters becomes effortless and efficient.
Hyperparameter Optimization Methods in Optuna
Optuna provides various hyperparameter optimization methods, such as TPE (Tree-structured Parzen Estimator) and random search. TPE is a Bayesian optimization algorithm that balances exploration and exploitation, while random search explores the search space randomly to find the best hyperparameters. With these optimization methods, Optuna enables efficient exploration of the hyperparameter search space, increasing the chances of finding the best hyperparameters. By combining different optimization methods, Optuna offers flexibility and versatility in the hyperparameter optimization process.
Traditional Methods
Traditional methods of hyperparameter optimization involve manual tuning, with grid search and random search being commonly used approaches. These methods often require a large number of trials to find optimal hyperparameters, making them time-consuming and computationally expensive. In contrast, modern tools like Optuna offer more efficient and effective alternatives for hyperparameter optimization, leveraging advanced algorithms and techniques to expedite the process and yield better results. By automating the search space exploration, Optuna reduces the burden of manual tuning and enables researchers to achieve optimal hyperparameters in less time.
Bayesian Method
The Bayesian method leverages prior knowledge to guide the search for optimal hyperparameters. By modeling the objective function and updating the model with each trial, Bayesian methods tend to be more efficient than traditional approaches. They can effectively handle noisy or uncertain evaluations of the objective function. Popular Bayesian optimization frameworks such as Gaussian processes and Bayesian neural networks enhance the hyperparameter optimization process.
Early Stopping Method
Early stopping is a technique that halts the optimization process when the objective function no longer improves. This approach can help save time and computational resources by terminating the search early. Moreover, it prevents overfitting and enhances generalization performance. Early stopping methods continuously monitor the evaluation score throughout the optimization process. Once the evaluation score reaches a plateau or starts to deteriorate, early stopping is triggered.
Evolutionary Method
Evolutionary methods, inspired by natural selection, utilize mechanisms like mutation, crossover, and selection to evolve hyperparameter configurations. These methods efficiently explore large search spaces, making them ideal for complex or non-linear problems. One popular example of evolutionary methods is genetic algorithms. With their ability to adapt and evolve, these methods offer a powerful approach to hyperparameter optimization. By leveraging the principles of evolution, researchers have achieved impressive results in various domains.
Optuna's Unique Strategies
Optuna offers a range of unique strategies for hyperparameter optimization. These strategies include various sampling methods, pruning mechanisms, and study objects. With Optuna's define-by-run API, it becomes easy to define the search space and objective function. Optuna supports both single-objective and multi-objective optimization, providing flexibility for different scenarios. Additionally, Optuna's study objects enable efficient tracking and visualization of the optimization history.
Sampling Strategy
Optuna employs different sampling strategies to determine how hyperparameter configurations are sampled. It supports two main strategies: random search and tree-structured Parzen estimator (TPE). Random search explores the search space randomly, while TPE focuses on promising areas. The choice of sampling strategy depends on the specific characteristics of the optimization problem. Additionally, Optuna allows for the flexibility to define custom sampling strategies to suit individual needs.
TPESampler - Tree-Structured Parzen Estimator
TPESampler, a sampling strategy in Optuna that is based on the Tree-Structured Parzen Estimator algorithm, utilizes Gaussian kernel functions to model the objective function. By balancing exploration and exploitation during the optimization process, TPESampler has demonstrated its effectiveness in finding good hyperparameter configurations. This particular sampling strategy is particularly useful for problems with a large search space. With TPESampler, users can optimize their hyperparameters efficiently, saving time and resources.
NSGAIISampler - Non-Dominated Sorting Genetic Algorithm II
NSGAIISampler, a sampling strategy in Optuna, is designed for multi-objective optimization problems. It utilizes non-dominated sorting and crowding distance to guide the search process, allowing it to handle multiple conflicting objectives and find a set of Pareto optimal solutions. NSGAIISampler is particularly useful for problems where a single optimal solution doesn't exist. With this algorithm, Optuna users can efficiently explore the search space and identify hyperparameter configurations that achieve optimal results.
Pruning in Optuna
Pruning in Optuna is a technique that helps to early terminate unpromising trials, saving time and computational resources. Optuna offers various pruning mechanisms like median pruning and asynchronous hyperband. These mechanisms monitor trial's intermediate results to decide whether to continue or prune. Pruning is particularly beneficial for optimization problems with long evaluation times, allowing researchers to focus on promising trials while discarding less promising ones.
Pruning Mechanism and Its Implementation in Optuna
Optuna implements efficient pruning mechanisms to save time and computational resources by early terminating unpromising trials. One such mechanism is the median pruning, which compares a trial's intermediate results with the median of other trials. If the intermediate result is worse than the median, the trial is pruned. Another mechanism is the asynchronous hyperband, which dynamically allocates computational resources to promising trials. These pruning mechanisms significantly enhance the efficiency of the optimization process.
SuccessiveHalvingPruner - Asynchronous Successive Halving
Optuna's SuccessiveHalvingPruner leverages asynchronous successive halving to efficiently prune unproductive trials during hyperparameter optimization. This method optimizes the search space and reduces computation time. By dynamically allocating computational resources to the most promising trials, Optuna maximizes efficiency. The implementation of SuccessiveHalvingPruner in Optuna results in faster and more efficient hyperparameter optimization, enabling researchers to achieve optimal results in less time.
Visual Interpretation with Optuna
Optuna offers a range of visualization functions that aid in interpreting and analyzing hyperparameter optimization outcomes. These visualizations, such as parameter importances and optimization history, provide insights into the impacts of different hyperparameters on the objective function. By gaining visibility into the optimization process, users can make informed decisions and better understand algorithm behavior. Optuna's visual interpretation features facilitate the identification of optimal hyperparameters for specific machine learning tasks, enhancing transparency and interpretability throughout the hyperparameter optimization process.
Documentation and Visualization Features in Optuna
Optuna provides comprehensive documentation that explains the functionalities and usage of the tool. The documentation includes detailed examples and code implementation guidelines for different use cases, helping users understand the various optimization methods and concepts. Additionally, Optuna's visualization features enable users to visually analyze the optimization process and results, enhancing the user experience and facilitating effective hyperparameter optimization.
Speed and Parallelization in Optuna
Optuna ensures efficient hyperparameter optimization through speed and parallelization. By supporting parallelization, it reduces overall optimization time by running multiple trials simultaneously. This makes Optuna suitable even for high-dimensional optimization problems. Additionally, Optuna allows users to leverage distributed computing environments for further speed improvements. With its speed and parallelization features, Optuna enables fast and effective hyperparameter search without compromising result quality.
How Fast is Optuna Compared to Other Tools?
Optuna's speed has been benchmarked against other hyperparameter optimization tools, consistently demonstrating faster convergence with fewer trials. Its efficient implementation, optimization algorithms like TPE and Random Search contribute to its rapid performance. This makes Optuna a top choice for quickly finding the best hyperparameters for machine learning models.
Hands-on With Optuna
Implementing Optuna is simple, requiring minimal code. Optuna offers code examples and tutorials to guide users through hyperparameter optimization. Users can define the objective function and search space easily with Optuna. Integration with existing machine learning frameworks is flexible, thanks to Optuna's Define-by-Run API. By gaining hands-on experience with Optuna, users can efficiently optimize their models and achieve better performance.
Code Implementation and Examples
Optuna provides comprehensive code implementation guidelines and examples for various use cases, allowing users to easily integrate it into their projects. By referring to these examples, users can gain a better understanding of how to implement Optuna in their own machine learning models. The code implementation guide offers step-by-step instructions for setting up and running hyperparameter optimization with Optuna, making it accessible even for those new to the tool. With a wide range of code examples available, users can quickly get started with Optuna and simplify the process of hyperparameter optimization.
What Makes Optuna the Ultimate Hyperparameter Optimization Tool?
Optuna stands out as the ultimate hyperparameter optimization tool due to its unique combination of speed, efficiency, and user-friendly interface. With advanced algorithms and parallelization capabilities, it delivers fast and accurate results. Documentation and visualization features enhance understanding, while hands-on implementation makes it easy to integrate into machine learning workflows.
KeywordSearch: SuperCharge Your Ad Audiences with AI
KeywordSearch has an AI Audience builder that helps you create the best ad audiences for YouTube & Google ads in seconds. In a just a few clicks, our AI algorithm analyzes your business, audience data, uncovers hidden patterns, and identifies the most relevant and high-performing audiences for your Google & YouTube Ad campaigns.
You can also use KeywordSearch to Discover the Best Keywords to rank your YouTube Videos, Websites with SEO & Even Discover Keywords for Google & YouTube Ads.
If you’re looking to SuperCharge Your Ad Audiences with AI - Sign up for KeywordSearch.com for a 5 Day Free Trial Today!
Conclusion
To sum it up, Optuna is a powerful and versatile hyperparameter optimization tool that offers a wide range of features and benefits. With its efficient architecture, it allows for easy implementation and evaluation of different optimization methods. The unique strategies and pruning mechanisms in Optuna enhance the search process and improve efficiency. Additionally, Optuna provides visual interpretation and documentation features that aid in understanding and analyzing the optimization results. Its speed and parallelization capabilities make it a top choice for hyperparameter optimization tasks. Whether you are a beginner or an experienced practitioner, Optuna offers code implementation and examples that make it accessible and user-friendly. With all these advantages, it's clear that Optuna stands out as the ultimate hyperparameter optimization tool.